Agentic AI
Agentic AI is a powerful evolution of AI that may empower organisations to operate more autonomously. It harnesses advanced forms of artificial intelligence, including Gen AI, in the form of agents that can be given personas that learn from data from other agents and adapt their behaviour over time. Agentic AI acts more like a partner than a tool, enabling enterprises to perform complex workflows and decision-making.
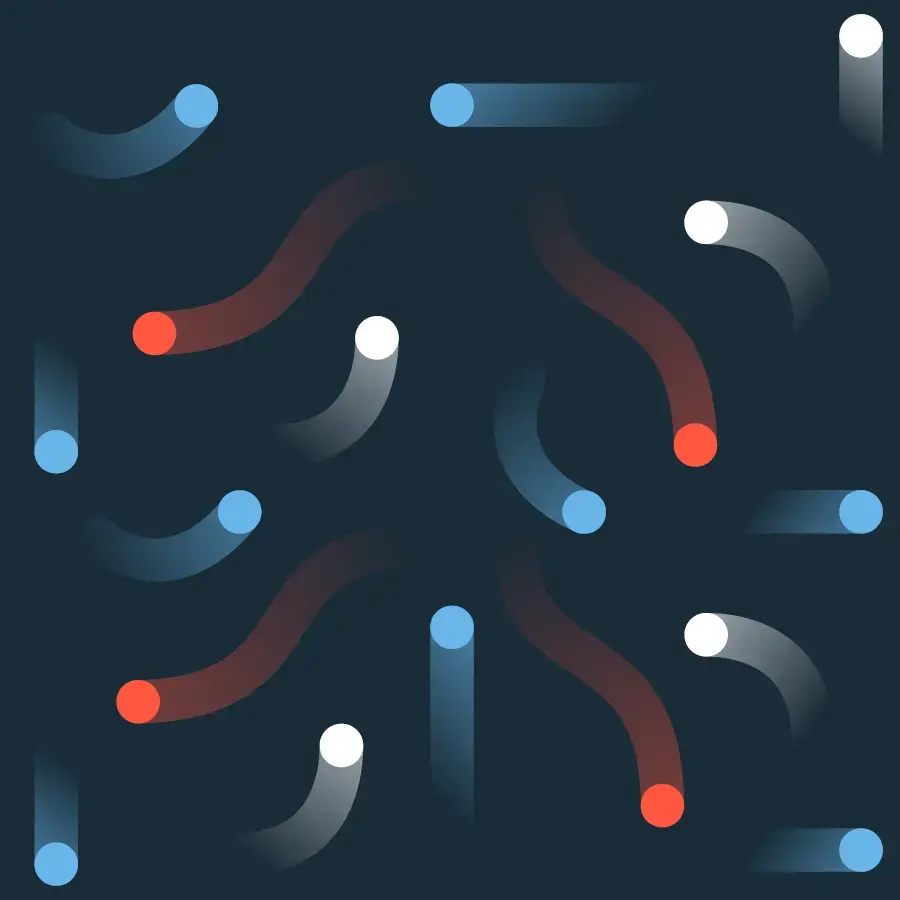
What is agentic AI?
Agentic AI is an artificial intelligence system with enhanced autonomy, decision-making capabilities and adaptability. These systems are designed to pursue complex goals and execute workflow tasks with limited direct human supervision.
Unlike traditional AI systems programmed for specific tasks, agentic AI can understand and interpret complex, nuanced contexts and goals, allowing for more specific interactions and decision-making processes based on well-defined workflows.
Agentic AI aims to operate more like a human employee, exhibiting agency characteristics to act autonomously and make choices. It's designed to understand context and instructions in natural language, set appropriate goals, reason through tests and adapt decisions and actions based on changing conditions.
This type of AI is often associated with more advanced forms of machine learning, Gen AI and cognitive computing, where algorithms can learn from data and adapt their behaviour over time.
Capabilities of agentic AI include (but are not limited to):
- Autonomy: The ability to act with limited human oversight.
- Reasoning: The ability to make judgement calls and weigh trade-offs with contextual decision-making.
- Reasoning: The ability to make judgement calls and weigh trade-offs with contextual decision-making.
- Language understanding: Comprehending and following natural language instructions.
- Workflow optimisation: Moving between subtasks and applications efficiently to complete processes.
Due to the complexity of processes and goals, agentic AI workflows are often used in larger enterprises.
Evolution of agentic AI
Agentic AI evolved from conversational AI and other variants. From simple chatbots, conversational AI evolved into intelligent AI copilots that can understand language and autonomously make decisions. These systems now interpret instructions more accurately, set subgoals to complete multi-step tasks and adjust to real-time developments to change course when needed. They are often aided by a human in the loop who may review their output and can help provide guidance where necessary.
Earlier iterations of conversational AI relied on basic pattern matching and prompted responses within pre-defined domains. However, these systems lacked deeper language comprehension and could only handle simple inquiries.
As these models have advanced, they've been trained on larger amounts of data, improving their response across various prompts. Enterprises use AI agents to manage complex goals and agentic workflows. This has driven demand for agentic capabilities like autonomous goal setting, reasoning, decision-making, language understanding and the ability to integrate with existing systems.
These agentic capabilities have led to new AI solutions, such as copilots, designed to operate with limited human oversight and enable reliable automation of complex tasks.
Agentic AI vs. traditional AI
While agentic AI is a type of AI, it differs from traditional AI.
Traditional AI operates primarily on specific algorithms using largely structured data and set rules. These models are designed to perform well-defined tasks, such as sorting data, facial recognition or language translation. The scope of traditional AI is limited to its programming and cannot deviate away from its given instructions or learn from new experiences.
On the other hand, agentic AI systems are designed to be more autonomous and adaptive. They process data and make decisions, learn from interactions and take proactive steps toward achieving complex goals by anticipating needs and suggesting actions. The idea is to give machines agency so they act more like partners than tools. The use of personas can be an important part of the success of agentic AI.
Agentic AI leverages innovations like:
- Large language models (LLMs) that comprehend nuanced human speech
- Scalable computing power to train complex models
- Very large datasets to enable deep learning
- The ability to connect and interact with other systems
Types of agents in AI
Agentic AI models run on autonomous agents. There are two main types of agents in agentic AI:
- Reactive: Agents that respond to immediate stimuli from their environment and take actions based on those stimuli.
- Proactive: Agents that take initiative and plan to achieve their goals.
The environment in which an agent operates can be fixed or dynamic. Fixed environments have static rules that do not change, while dynamic environments constantly change and require agents to adapt to new scenarios.
Agentic AI applications
Autonomous agents are used in a wide range of AI applications. They can be implemented using different programming languages and techniques, including machine learning and natural language processing.
A few agentic AI examples include:
- Smart homes and buildings: Agents can control heating, lighting and other systems in smart homes and buildings, optimising energy use and improving comfort.
- Smart homes and buildings: Agents can control heating, lighting and other systems in smart homes and buildings, optimising energy use and improving comfort.
- Healthcare: Agents can monitor patients, provide personalised treatment plans and optimise healthcare resource allocation.
- Finance: Agents can automate trading, fraud detection and risk management in the financial industry.
- Games: Agents can create intelligent opponents in games and simulations, providing players with a more challenging and realistic experience.
- Natural language processing: Agents can translate languages, answer questions and operate chatbots to communicate with users in their natural language.
- Cybersecurity: Agents can detect intrusions, analyse malware and monitor network security.
- Environmental monitoring: Agents can monitor and manage natural resources, track climate change and improve environmental sustainability.
- Social media: Agents can analyse social media data, identify trends and patterns and provide personalised recommendations to users.
- Intelligent personal assistants: Agents can help users with various tasks, such as scheduling appointments, sending messages and setting reminders.
Business impact of agentic AI
Agentic AI promises to unlock new benefits for businesses, including increased efficiency, improved decision-making and optimised operations. It can help enterprise businesses automate complex workflows and repetitive tasks and also perform real-time analysis of large data volumes to support the decision-making process. Agentic AI also helps organisations optimise their operations by dynamically responding to shifting conditions to help minimise costs.
Moreover, agentic AI helps businesses enhance their customer experience by developing personalised recommendations and customer interactions. It can also aid in predictive maintenance, helping companies monitor their equipment and assets to manage risk, reduce downtime and alleviate maintenance costs.
Businesses can also use agentic AI in their innovation and product development efforts by analysing market trends, customer feedback and competitor data to identify new opportunities.
Agentic AI offers powerful capabilities for enterprises across industries to aid in proactive decision-making. It provides organisations with autonomy to drive efficiency and optimised operations. From personalised customer experiences to fraud detection, agentic’s AI services applications are limitless.
Further reading
Check out these resources for more information about how to use agentic AI in people-centric innovation.