Automotive Data
From real-time performance metrics to driver-behaviour insights, the data generated by modern vehicles is revolutionising the automotive industry. With connected cars and IoT devices on the rise, automotive data is becoming a cornerstone for innovation, helping manufacturers, drivers and service providers optimise every aspect of mobility.
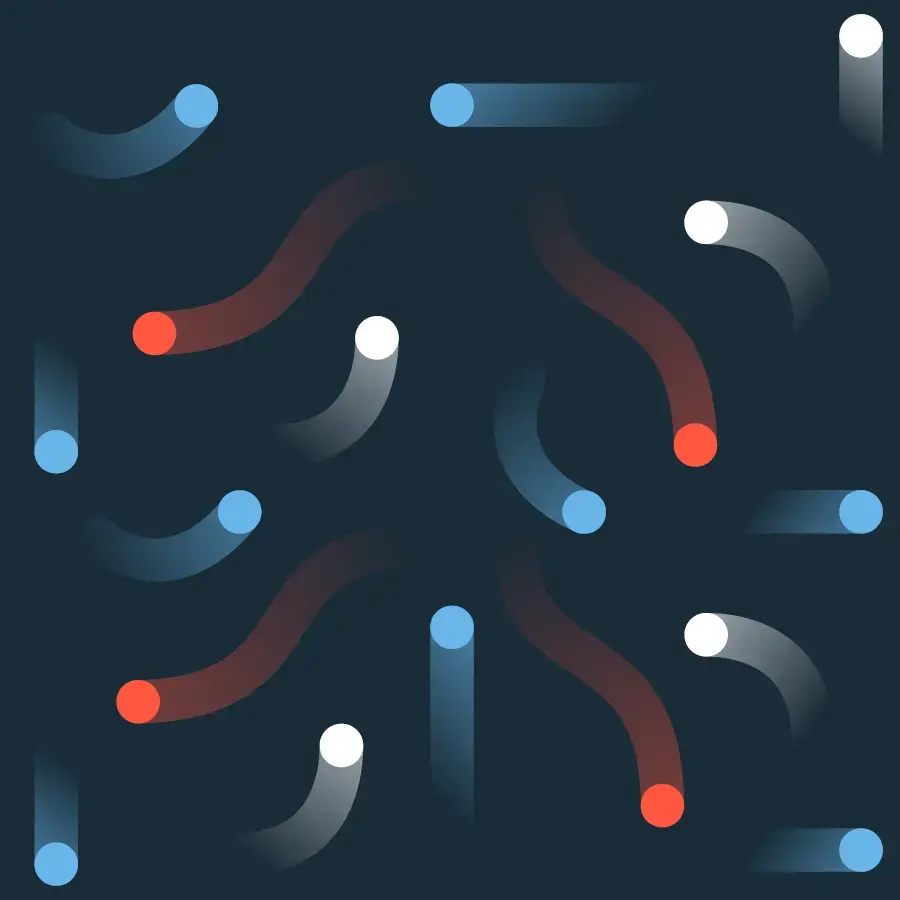
What is automotive data?
Automotive data is information about a vehicle's operation, performance and usage collected by sensors and systems.
Automotive data can be used to improve:
- Vehicle performance and safety
- Product design and provide predictive maintenance
- The driving experience
- Risk assessment and pricing models
It includes data points such as vehicle specifications, maintenance records, vehicle history reports, fuel consumption data and telematics. This data type is used for vehicle research, market analysis, predictive maintenance, insurance underwriting and fleet management while providing insight into driver behaviour and vehicle health.
Automotive data plays an important role in developing advanced features like autonomous driving, connected cars, predictive maintenance and personalised in-car experiences.
Types of automotive data
Motor vehicles contain a wealth of information. The type of data generated depends on the vehicle brand and even within brands, from model to model. Automotive data can be broadly categorised into the following types:
Vehicle data
Vehicle data can be divided into operational, telematics and advanced driver assistance system (ADAS) data. Operational data pertains to the vehicle's performance, (i.e., speed, fuel efficiency, and engine diagnostics). Telematics data includes data points such as GPS location, driving patterns and remote diagnostics. Finally, ADAS data is input from cameras, radar and LIDAR sensors.
Driver data
Organisations can collect behaviour data from drivers. This includes insights into their driving habits, such as braking patterns, acceleration and adherence to speed limits. Additionally, driver preferences such as seat positions, climate control and infotainment choices can be collected.
Environmental data
Data about driving conditions can also be collected. This includes road data, information about road conditions, traffic, hazards, and weather data that affect driving, such as temperature or precipitation.
Business and customer data
Lastly, cars offer a wealth of business and customer data. Maintenance data can provide insight into service records, warranties and repair history. Sales data can share information about purchases, financing and leasing. Lastly, customer feedback data can help better understand ratings, reviews and other user satisfaction metrics.
Sources of automotive data
Common automotive data can be sourced from:
- Onboard sensors: Cameras, accelerometers and gyroscopes
- Telematics systems: Data transmitted from external servers via cellular or satellite connections
- Connected devices: Smartphones, wearables and other devices paired with the vehicle
- Third-party services: Apps and platforms that provide navigation, streaming and insurance services.
- Maintenance shops and dealerships: This data is generated during inspections, repairs and vehicle purchases.
Applications of automotive data
Automotive data has a wide range of applications across industries:
- Vehicle development to improve car design, safety and performance based on real-world data
- Predictive maintenance to anticipate failures and schedule repairs to reduce downtime
- Autonomous driving that enables self-driving systems by training AI on large datasets from sensors and road conditions
- Personalised driving experiences that tailor in-car settings and infotainment options to individual preferences
- Fleet management to optimise routes, reduce fuel costs and monitor vehicle health for commercial fleets
- Insurance that powers usage-based insurance models that assess premiums based on driving behaviour
- Urban planning to inform infrastructure projects with traffic and road usage data
Automotive data technologies
Automotive data relies on an ecosystem of technologies to collect, process, analyse and apply insights. Here are some critical technologies behind automotive data:
Internet of Things (IoT)
IoT connects vehicles with external devices and systems, enabling seamless data exchange. Sensors collect data on speed, fuel usage, engine performance and tyre pressure. IoT also enables vehicle-to-vehicle (V2V) and vehicle-to-infrastructure (V2I) communication to improve traffic flow.
Cloud computing
Cloud computing provides scalable storage and computing power for managing large volumes of automotive data. Vehicle data is transmitted to the cloud for analysis and long-term storage. Cloud computing also facilitates over-the-air (OTA) updates to vehicle software.
Edge computing
Edge computing processes data within the vehicle to reduce latency. It supports real-time decision-making in ADAS and enables low-latency applications like collision detection.
Artificial intelligence (AI) and machine learning (ML)
AI and ML analyse and interpret complex automotive data to enable intelligent decision-making. It powers self-driving algorithms that process sensor data for navigation. AI and ML can also aid in personalising driver experiences by learning preferences.
5G connectivity
5G connectivity offers high-speed, low-latency connectivity critical for real-time applications. It enables vehicle-to-everything (V2X) communication for smart city integration and improves data transfer rates for in-car entertainment and telematics.
Advanced analytics
Advanced automotive analytics allows for processing massive datasets to uncover trends and actionable insights. It can help identify patterns in driver behaviour to optimise fuel efficiency. Additionally, it gives insight into predictive maintenance based on historical and real-time data.
Digital twins
Digital twins create virtual replicas of vehicles and systems for simulation and testing. They help optimise vehicle design and performance before physical production. Digital twins can also enable predictive maintenance by simulating wear-and-tear scenarios.
Benefits of automotive data
Automotive data is important for several reasons, including:
- Improving vehicle safety, reliability and performance. Automotive data provides insights into how vehicles are used and performed in different conditions and when maintenance is required. This can help manufacturers to improve their products and enhance the overall customer experience.
- Personalising marketing and sales efforts. Automotive data enables companies to personalise their offerings and target specific customer segments.
- Optimising supply chain management and logistics. Automotive data allows manufacturers to optimise production processes and manage inventory levels more efficiently, which can help reduce costs and improve overall efficiency.
- Automotive data enables innovation and new business models. It helps companies identify new business opportunities and develop innovative products and services.
Businesses can use automotive data to enhance vehicle performance, improve safety and deliver tailored customer services. Through automotive industry consulting, organisations can strategically harness automotive data to shape the future of mobility, ensuring smarter, safer and more efficient driving experiences.
Further reading
Check out these resources to learn more about automotive data and its role in people-centric innovation.