Generative AI (Gen AI)
Generative AI is a groundbreaking field at the forefront of artificial intelligence. It harnesses sophisticated algorithms to create new data samples, including images, music, text and simulations. This innovative technology is revolutionising industries and reshaping the future of AI-driven solutions across various domains to create novel content, solve complex problems and drive innovation in the real world.
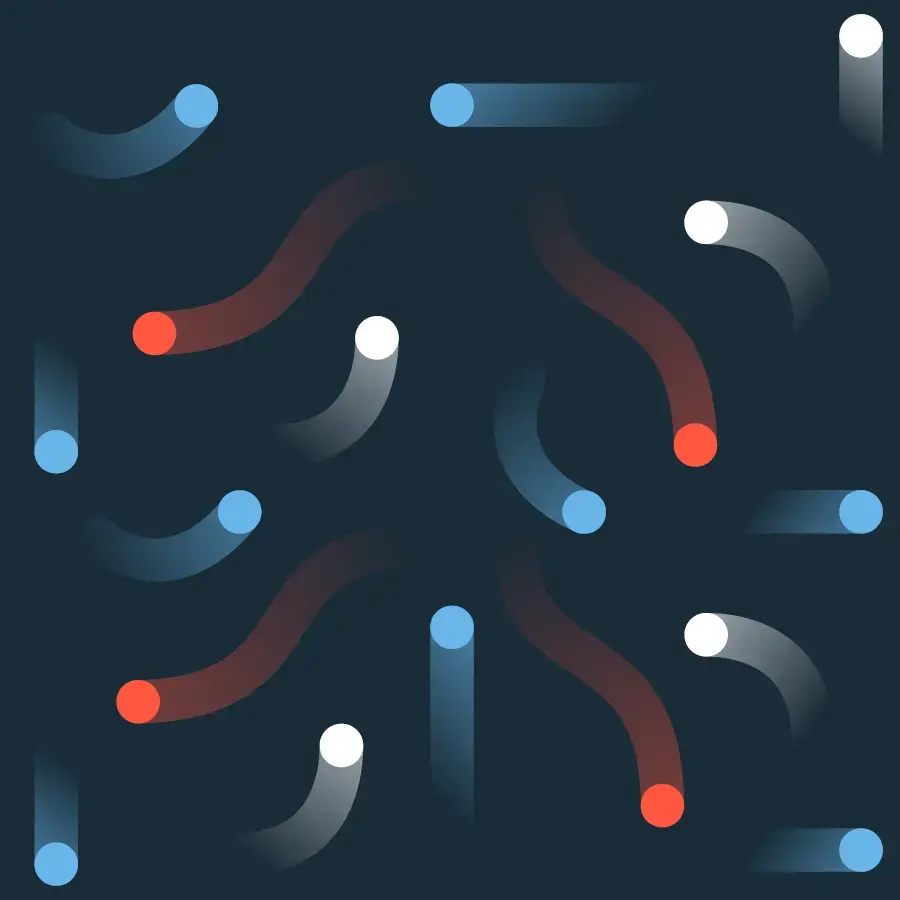
What is generative AI?
Generative AI is a branch of AI focused on creating models to produce new data samples resembling those in a given dataset. It utilises deep learning and large language models (LLMs) to understand patterns and structures, enabling fresh content generation. Unlike traditional AI, which focuses on classification or prediction, generative AI seeks to replicate the underlying data patterns to create new data.
Generative AI has applications across various industries beyond language generation, including image, music, art and synthetic data generation.
A few examples of gen AI are Google’s DeepDream, which generates psychedelic images based on neural network algorithms, and Open AI’s ChatGPT, which generates human-like text. In the fashion industry, tools like Zeekit and Vue.ai use generative AI to allow people to try on clothes virtually before purchasing. Other gen AI companies, such as Atomwise and Insilico Medicine, use generative AI for drug discovery research.
Advancements in generative AI are rapid thanks to ongoing, boundary-pushing research. These models could revolutionise human creativity, productivity and interaction with technology.
History of AI
While generative AI is a recent technology, the concept of AI dates back at least 70 years when humans wondered if machines could think and process like humans.
The dawn of AI began in the 1950s when researchers were exploring the possibilities of machines that could simulate human intelligence. This started with text analytics, primarily focusing on information retrieval and keyword extraction tasks. The idea was for computers to understand and manipulate text in a way that mirrored human comprehension.
A decade later, AI research shifted toward rule-based systems and knowledge bases, which encode human knowledge and expertise into computer programs using rules and reasoning. This led to the development of expert systems that could solve specific problems by following predefined rules.
Natural language processing (NLP) emerged in the 1980s and 1990s, enabling machines to understand and generate human language. AI researchers began to develop more advanced techniques for parsing and analysing text, allowing applications like machine translation, speech recognition and sentiment analysis.
The 2000s saw a rise in machine learning due to the vast amount of data available and the breakthrough of cloud computing which has high levels of processing power. Machine learning algorithms, particularly neural networks, proved highly capable of understanding and generating human language.
This leads us to the 2020s and GPT-3 (Generative Pre-Trained Transformer 3). GPT-3 was the launch point for generative AI, pre-trained on an enormous amount of text data and able to generate coherent and contextually relevant text. We now have GPT-4, and GPT-5 is expected to launch in the next 6-12 months.
Potential of generative AI for business transformation
By strategically leveraging AI, businesses can unlock new opportunities, improve operational efficiency and gain a competitive advantage in the marketplace. Deloitte estimates that the market for gen AI will grow to $200 billion by 2032, representing about 20% of total AI spend.
AI offers companies a transformative toolset for business evolution across various fronts. From streamlining product design and prototyping processes to enabling immersive virtual try-on experiences in industries like fashion and interior design, gen AI can help enhance customer engagements and may help accelerate decision-making. Generative AI also helps businesses aid in their creative processes to generate new content, create realistic simulations and provide content that can be used to train new models.
Moreover, it addresses data scarcity challenges by synthesising realistic anonymous data sets that can be used in industries like healthcare and finance while refining recommendation systems to deliver personalised suggestions tailored to individual preferences, thereby boosting customer satisfaction and sales.
Through these applications, gen AI empowers businesses to innovate, streamline processes and cultivate deeper customer relationships. As the technology evolves, its potential for business transformation continues to expand, paving the way for further advancements and opportunities.
Types of generative AI models
Gen AI models are a subset of AI models designed to generate new data such as following patterns found in existing data. Many of these models use unsupervised learning techniques where the model learns patterns and structures in data without explicit labels or targets, allowing it to generate data without specific requirements. Lastly, generative models produce diverse outputs.
There are several gen AI models:
Generative Adversarial Networks (GANs)
GANs are used for image generation, style transfer and content creation. They consist of two neural networks: a generator and a discriminator. The generator creates data while the discriminator evaluates its authenticity, improving its ability to create realistic data.
Variational Autoencoders (VAEs)
VAEs operate on the principles of probabilistic modelling for image generation, data compression and image reconstruction. They aim to learn about the underlying probabilistic distribution of the data.
Recurrent Neural Networks (RNNs)
RNNs are neural networks specifically designed for sequential data, such as time series data. They are primarily used for text generation, machine translation and speech recognition.
Long Short-Term Memory (LSTM) Networks
LSTMs are a specialised type of RNN that captures long-range dependencies in sequential data. They are the most effective in NLP tasks, including language modelling, text generation and sentiment analysis.
Generative Pre-trained Transformers (GPT)
GPT models leverage transformer architecture and large-scale pre-training on text data to generate text. They are primarily used for natural language understanding and generational tasks, including chatbots, content generation, translation and more.
Transformers
Transformers are neural networks that use deep learning architecture to predict new text based on sequential data. They can learn context and “transform” one type of input into a different type of output to generate human-like text and answer questions.
Generative AI vs. predictive AI vs. LLMs: What is the difference?
Gen AI, predictive AI and Large Language Models (LLMs) are all subsets of AI, but they serve different purposes and operate differently.
Generative AI
Gen AI generates new data that resembles those in a given dataset. Generative AI aims to understand and replicate the structures and patterns present in the data to create new samples.
Gen AI uses various models to generate data by learning from actual data samples or creating representations of a probability distribution that generates the data.
It can also be applied to image generation, text synthesis, music composition, data augmentation and more.
Predictive AI
Predictive AI primarily predicts future events or outcomes based on historical data. It uses various machine learning algorithms, such as regression, classification and time-series forecasting models to analyse data patterns and make informed predictions about what will happen next.
These models learn from labels or historical data to predict unseen or future data.
Common use cases for predictive AI include financial forecasting, demand forecasting, risk assessment, healthcare diagnosis, recommendation systems, predictive maintenance and resource-allocation optimisation.
LLMs
LLMs primarily focus on understanding and generating human-like text. They excel at language translation, text summarisation, sentiment analysis, answering questions and text generation.
LLMs are typically based on deep learning architectures, particularly transformer-based architectures. They are trained on large text datasets, learning language patterns, grammar, context and semantics.
LLMs are commonly used for NLP tasks, including chatbots, virtual assistants, content generation and more.
Generative AI applications
Gen AI presents significant opportunities for financial services, spanning operational efficiency improvements, enhanced customer experiences, risk mitigation and fraud prevention. Key use cases include market simulation for strategy testing, fraud detection enhancement, personalised customer service and compliance support.
Gen AI revolutionises diagnosis accuracy, treatment personalisation, drug discovery and patient care in healthcare. It augments datasets with synthetic medical images, accelerates drug discovery through molecule design, automates medical report generation and tailors treatment plans based on patient data.
Gen AI facilitates personalised learning, instructional efficiency and access to quality education resources. It designs tailored educational content, aids language learning with synthetic exercises, powers virtual tutoring systems, creates immersive learning experiences, automates assessments and summarises educational materials.
Generative AI in finance and banking
Gen AI gives banks many opportunities to enhance operational efficiency, elevate customer experiences, manage risks and combat financial fraud.
Through capabilities like market simulation, fraud detection, portfolio management, algorithmic trading support, personalised customer service, Anti Money Laundering (AML) compliance and credit scoring and risk assessment, Gen AI empowers financial institutions to optimise strategies, detect and prevent fraudulent activities, tailor services to individual clients and navigate complex regulatory landscapes.
By leveraging Gen AI technologies, banks can foster innovation, improve decision-making processes and bolster trust and security within the financial ecosystem.
Generative AI in healthcare
AI stands poised to revolutionise healthcare, promising more accurate diagnoses, personalised treatments and efficient drug discovery processes.
Gen AI addresses critical challenges in healthcare, from data scarcity to treatment optimisation through medical image generation, drug molecule design and personalised treatment planning.
Moreover, its applications extend to medical text generation for streamlined documentation, medical education through realistic simulations and healthcare chatbots for personalised patient interaction, ultimately advancing patient care, medical training and clinical efficiency.
Generative AI in education
Gen AI can revolutionise education by fostering personalised learning experiences, enhancing instructional efficiency and broadening access to high-quality educational materials.
Through capabilities like curriculum design, personalised learning plans, virtual tutoring, immersive learning experiences, automated assessments and content summarisation, Gen AI empowers educators to tailor instruction to individual student needs, facilitate interactive learning and provide timely feedback.
By leveraging gen AI tools, educators can create engaging and effective learning environments that cater to diverse learning styles, ultimately enriching the educational experience for students and educators alike.
Limitations of generative AI
While generative AI boosts several benefits, it also has several limitations:
- Biased, outdated or unreliable information. Since Gen AI creates content based on data it has been trained on, the information could be biased, outdated or unreliable. Hence, vetting and validating output is essential to ensure they’re pulling reliable information.
- Generative AI hallucinations. While AI models generate new content based on facts, they can sometimes add their creative interpretation, resulting in distorted information. This is what’s known as an AI hallucination, where the AI model generates false content based on their understanding of a scenario or context.
- Lack of creativity. While it can mimic creative styles, Gen AI lacks true creativity, imagination, emotional depth and critical thinking since it relies on patterns and historical data rather than pure inspiration.
- Concerns about human replacement. AI technology is meant to enhance productivity and skills, but sceptics fear that it could lead to human replacement. According to McKinsey, generative AI will compete with the top 25% of people, completing any tasks before 2040.
- Ethical and privacy concerns. Gen AI raises ethical considerations regarding generating synthetic data, potential misuse of generated content, and privacy implications. There are concerns about the unintended consequences of generating highly realistic deepfake videos or text that could be used for malicious purposes.
Gen AI has emerged as a transformative force across industries, driving innovation and reshaping traditional paradigms. From revolutionising product design to personalising customer experiences, gen AI offers many possibilities for businesses to thrive in the digital era. As AI solutions like it continue to evolve, its potential for business transformation remains boundless, offering a pathway to enhanced efficiency, deeper insights and unparalleled creativity.
Further reading
Check out these resources to learn more about generative AI and its role in people-centric innovation.