Scaling AI
AI has emerged as a transformative force, revolutionising industries and redefining organisations' operations. The rapid growth of AI and machine learning (ML) is a testament to their potential to drive innovation, boost efficiency and unlock new revenue streams. But first, organisations need to scale it effectively.
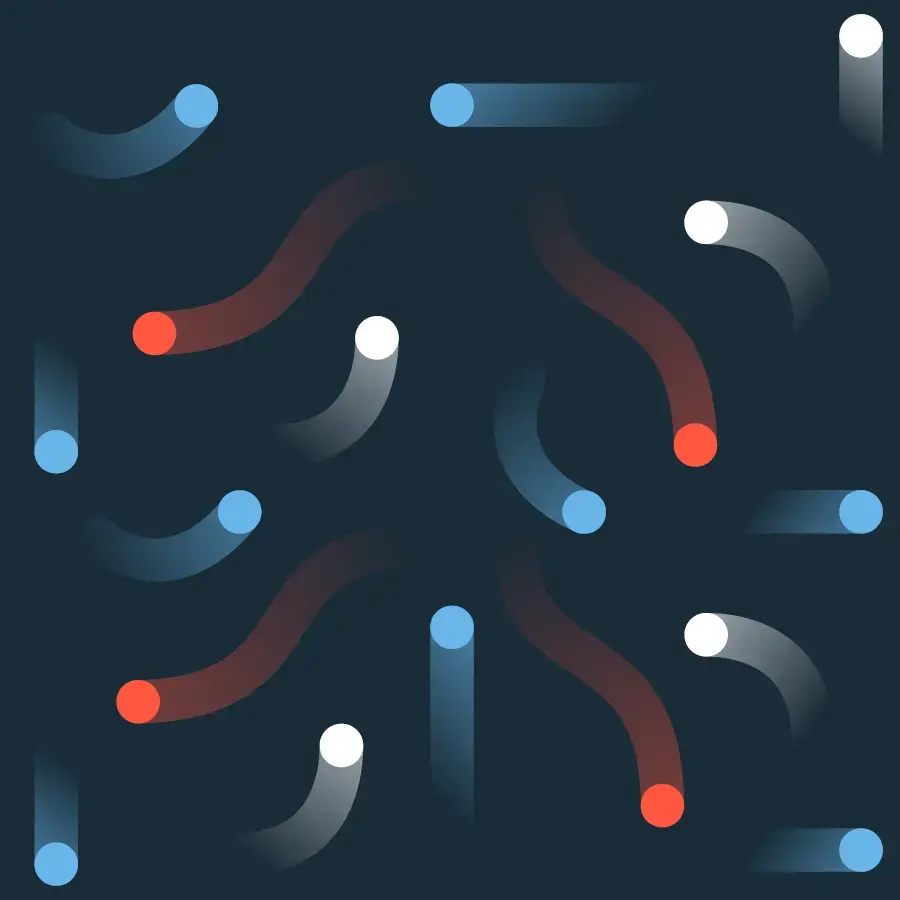
What does it mean to scale AI?
Scaling AI means integrating AI technologies across the business to enhance processes, increase efficiency and drive growth while managing risk and elevating compliance. It may involve, for example expanding the use of machine learning (ML) and AI algorithms to perform day-to-day tasks efficiently and effectively, matching the pace of business demand. I could also involve the use of Gen AI to increase the productivity of staff.
To achieve AI at scale, AI systems require robust infrastructure and substantial data volumes to maintain speed and scale. They rely on the integration and completeness of high-quality data from different parts of the business to provide algorithms with the comprehensive information necessary to achieve desired results.
AI is most valuable when operationalised at scale. AI at scale has moved beyond digitally native companies to various industries, such as manufacturing, finance and healthcare. As companies accelerate their adoption of AI technologies, they progress from isolated AI projects to full digital transformation.
Challenges for scaling AI
Many organisations struggle to maintain momentum as they scale their AI efforts. They often face challenges that lead to longer delivery timelines. While many organisations can successfully conduct experiments and create proof-of-concept models, they have trouble transitioning them to production-ready models.
When progressing from pilot projects to scaled AI initiatives, organisations need to determine an appropriate scope to avoid significant disruption. These projects, due to their complexity, take time to build and deploy. Time and effort must be dedicated to acquiring, integrating, and preparing data and monitoring AI outputs.
Organisations also need to ensure that they maintain high standards of security, regulatory and ethical compliance. Data teams may also struggle with productivity due to increasing complexity, inefficient collaboration and a lack of standardised procedures and tools.
Other complexities and challenges arise, such as potential performance degradation and limited visibility into AI model behaviour. Additionally, as data volumes continue to expand exponentially for training, testing and refining AI models, they must prioritise governance and security as they do so.
Scaling AI involves an iterative process that requires collaboration across multiple teams within an organisation, including business experts, IT and data scientists. It also means selecting the appropriate tools to build models and manage data, computing resources and tools for business users to interact with AI outputs.
By addressing these challenges, organisations can navigate the complexities of scaling AI and maximise its potential to improve operations and drive business value.
MLOps (machine learning operations)
To fully leverage their ML investment, organisations can introduce MLOps technology that efficiently transitions ML models from experimentation to production and facilitates ongoing maintenance and productivity once these models are deployed.
MLOps aims to establish best practices and tools for rapid, safe and efficient AI development, deployment and adaptability. It is the foundation for successful AI scalability and requires strategic investments in processes, people and tools to enhance speed-to-market while maintaining control over deployment.
MLOps ensures ML models are robust and efficient before deployment by automating key tasks, facilitating collaboration between teams and providing robust deployment pipelines and monitoring mechanisms. MLOps prevents ML models from becoming obsolete or unused due to degradation or drifts and offers a solution for continuously monitoring and improving them.
Scaling AI agents
Scaling AI agents is like having a supercharged human collaborator that may outperform individual capabilities and can embody multiple perspectives simultaneously. These agents don’t just multitask; they adopt different personas to thoroughly explore every facet of a problem before proposing the most optimised solution.
Their ability to absorb feedback, recalibrate, and pivot makes them incredibly flexible and adaptive. They handle immense workloads at speeds no human can match. Yet, AI agents aren't just robotic assistants; they're strategic partners and trusted advisors. They can collect, process, and distil data into actionable insights, guiding decisions with minimal input required from their human counterparts.
Rather than replacing humans, these AI agents enhance our capacity to focus on high-impact, strategic work, liberating us from the execution of repetitive, time-intensive tasks. However, human influence throughout the development and implementation process ensures we understand how they function, enabling us to guide and train them to deliver consistent, predictable outcomes. The goal is for these AI agents to operate alongside us, elevating productivity and driving innovation.
Scaling AI teams
LLM- and cloud-agnostic approach enables an entirely new way of thinking about autonomous teams, removing constraints and freeing up valuable people inside a business to do more meaningful work. In a multi-agent system, groups of agents work together to accomplish more than what a single agent can do alone – handling more complex challenges and workflows.
On a multi-agent autonomous team, a lead agent receives an instruction set and creates smaller chunks of activity to be distributed among specialist agents working on its behalf. As the pieces are completed, the lead agent orchestrates them back into the proper output and sends them to the next workflow step or a person for human review.
In the real world, when one team is overloaded, you call in extra help for support. Multi-agent systems leverage a similar mechanism to create multiple channels for high-intensity processes and move data through the pipeline more efficiently. Rather than having one agent grind out a sequential process, holding up other aspects that could move forward, the scale factor allows for a more streamlined approach to workflow throughput.
Considerations for scaling AI
As companies are looking to scale AI, there are four considerations to keep in mind:
- Interoperability. Your organisation probably already has some AI infrastructure in place. Choose a tool that will interoperate with the existing ecosystem to reduce friction or can be extended to support scaling efforts.
- Meet operator needs. To enable data scientists to do their best work, a platform must offer them flexibility to work independently. On the other hand, IT also needs a platform that imposes constraints and ensures that production deployments follow predefined and IT-approved paths. The best MLOps platforms can do both.
- Collaboration. An MLOps tool must make it easy for both data scientists to work with engineers and vice versa, for both personas to work with governance and compliance. While the speed of collaboration determines speed to market, governance collaboration ensures that the product being built is the one that should be built at all.
- Governance. With AI and ML, governance becomes more critical than in other applications. It's not just limited to an application’s security or access control, but also responsible for ensuring that an application is aligned with an organisation's ethical code, isn't biased toward one group, and that decisions made by the application can be trusted.
Scaling AI effectively allows organisations to achieve faster speed to market and lower costs. It allows them to maximise the potential of AI technology solutions and unlock new business value. Scaling AI gives organisations the ability to find new use cases and applications beyond what was previously imagined.
Further reading
Check out these resources to learn more about scaling AI and its role in people-centric innovation.